About
Participants of the educational program are offered a practice-oriented course, which is aimed at helping students acquire knowledge, skills and abilities to work with transcriptome data. The course consists of four sections: introduction to the discipline, study of the mechanisms of formation of RNA molecules, analysis of the structural organization of the transcriptome, as well as its functional annotation. These sections cover fifteen fundamental topics ranging from fundamental questions of transcriptomics to the use of transcriptomic data in predicting the survival of people with various diseases. Each course topic includes a relevant theoretical part and practical work. In addition, a series of self-study assignments are offered for each topic, as well as comprehensive advisory assistance.
Educational program
The program consists of fifteen topics, grouped into four sections. The study of each topic includes one lecture (2 hours), one or two practical exercises (from 2 to 4 hours, respectively), as well as independent work. One week is allotted for the study of each topic, however, changes in the schedule are possible at the request of the students. The overall training plan is presented below.
Week 1: Fundamental transcriptomics.
Introduction to the discipline. Transcriptomics as an integral part of modern molecular biology. Object and subject of research in transcriptomics. Transcriptome organization, structural and functional diversity of RNA molecules. Transcription and processing of RNA molecules, nuclear-cytoplasmic export/import of RNA molecules. The dynamics of the transcriptome.
Week 2: Experimental methods of transcriptomics.
Typical study design in transcriptomics. Input genetic material: type, quantitative and qualitative characteristics, biological and technical repeats. Classical methods of molecular biology in transcriptomics. High-performance technologies for generating transcriptomic data. Basic data formats. Assessment of the quality of primary data. Preprocessing and postprocessing of data. Guidelines and standards in transcriptomics.
Week 3: Mapping of short reads.
Data in FASTQ format. Sequencing quality assessment, pre-processing and filtering of primary data. Mapping short reads. Mapping algorithms. Local and global mapping. Mapping quality control. Survey of software solutions. Data in SAM/BAM format.
Week 4: Motif analysis.
DNA and RNA binding proteins. Binding site search algorithms. Data preparation. Motifs, consensus sequences and logos. Frequency, probability and weight matrices. Search for binding sites by motif matrices. Validation of analysis results.
Week 5: Identification and annotation of small capped RNAs.
Transcription mechanisms. Small capped RNAs, generation mechanism and biological role(-s). Methods for detection of small capped RNAs. Pre-processing, filtering, normalization and transformation of primary data. Pipeline. Annotation of identified small capped RNAs. Visualization and interpretation of the results of the analysis.
Week 6: Evaluation of the rate of RNA polymerase II.
Overview of methods for assessing the rate of RNA polymerase II. Assessment of the quality of primary data, their pre-processing and filtering. Mapping and summarizing short reads. Filtering, normalization and transformation of data. Hidden Markov models and their use to identify “waves” of RNA polymerase II activity. Calculation of the rate of RNA polymerase II. Visualization and interpretation of the results of the analysis.
Week 7: Identification and annotation of polyadenylation sites.
Transcription termination mechanisms in eukaryotes. Polyadenylation core, its structure and activity. Pre-processing, filtering, normalization and transformation of primary data. Overview of polyadenylation site identification algorithms. Pipeline. Annotation of identified polyadenylation sites. Visualization and interpretation of the results of the analysis.
Week 8: Gene expression analysis.
Basic pipeline. The study of gene expression at the level of individual transcripts or the whole gene, the advantages and disadvantages of each of the approaches. Pre-processing, filtering, normalization and transformation of raw data. Heteroscedasticity of data, stabilization of variability, elimination of the batch effect. Analysis of differential gene expression, models, software solutions. Visualization and interpretation of the results of the analysis.
Week 9: Transcriptome assembly.
Formalization of the transcriptome assembly problem based on transcriptome sequencing data. De novo assembly: an overview of algorithms and software solutions, advantages and disadvantages. Assembly based on reference annotations: sources of annotations, an overview of algorithms and software solutions, advantages and disadvantages. Assessing the quality of transcriptome assembly. Transcriptome annotation. GTF/GFF file format for storing transcriptome assemblies and annotations.
Week 10: RNA splicing analysis.
RNA splicing, a brief introduction. Detection of splicing events based on transcriptomic sequencing data. Study of splicing at the level of exons, exon-exon junctions and full-length transcripts. Differential usage of exons and exon-exon junctions. Models, algorithms, software solutions, advantages and disadvantages. Annotation of splicing events. Visualization and interpretation of the results of the analysis.
Week 11: Estimation of the coding potential of RNA molecules.
Structure of coding RNA. Identification of open reading frames. Structural and functional annotation of coding RNAs. Small and long non-coding RNAs, their identification by transcriptomic sequencing. Models, algorithms, software solutions, advantages and disadvantages. Structural and functional annotation of non-coding RNAs.
Week 12: Analysis of cellular molecular networks.
Molecular networks of the cell. Graph theory in molecular biology. Splicing graphs: definition, types, algorithms for reconstruction and quality assessment. Co-expression gene networks: types, algorithms for reconstruction and quality assessment. Gene regulatory networks: types, algorithms for reconstruction and quality assessment. Reference molecular networks. Modular networking. Topological analysis of networks. Biological interpretation of network topology.
Week 13: Analysis of the molecular pathways of the cell.
Organization and diversity of molecular pathways. Major databases on molecular pathways. Review of algorithms and software solutions in the field of molecular pathway analysis. Basic pipeline. Visualization of molecular pathways. Biological interpretation of molecular pathway analysis results.
Week 14: Gene ontologies.
The concept of gene ontologies. Type of gene ontologies. Structure of gene ontologies. Basic concepts and terms. Enrichment analysis. Gene set enrichment analysis. Models, algorithms, software solutions. Visualization and biological interpretation of the results of the analysis.
Week 15: Survival analysis.
Survival of patients as an integral indicator of the structural and functional state of the transcriptome of human cells. Preparation of clinical data, as well as data on the expression of the studied genes. Clinical outcome options. Filtering, normalization and transformation of primary data. Calculation of parameters of univariate and multivariate Cox proportional hazard regression models. Generalization of the statistical characteristics of the model. Graphical visualization of analysis results using the Kaplan-Meier curve. Interpretation of the results of the analysis.
Enrollment
Enrollment to the course is based on the results of an interview. The key selection factor is the applicant’s own motivation. At the same time, it should be noted that the educational program of the course is designed in such a way that the student does not need any special knowledge in the field of transcriptomics. However, user knowledge of computer technology as well as basic programming skills in R and/or Python are welcome. In addition, the condition of official enrollment in the course is the conclusion of a contract of the established form.
Form, place and time
The training program can be implemented:
- face-to-face;
- using information and communication technologies (remote conferencing such as Zoom);
- in a mixed form (part of the classes are face-to-face, some are using information and communication technologies);
- on-line (using video lectures, video tutorials and self-study activities; this form of learning is under development).
Classes can be held both in the daytime and in the evening by agreement with the students. The choice of the form of education is also consistent with the desires of the students. All forms of conducting classes include, if necessary, individual and group consultations, which can be held both in the classroom and remotely.
Classes are held on the basis of the State Educational Institution “Institute for Advanced Studies and Retraining in the Field of Informatization and Management Technologies” at Belarusian State University.
Course venue: Institute for Advanced Studies and Retraining of Belarusian State University, st. Kalvariyskaya-9, room. 730, Minsk, Belarus.
Time:
- day time: maximum 5 days a week – 8 academic hours per day (academic hour – 40 minutes) from 9:00 to 18:00. The optimal is 4 academic hours two or three times a week. The exact time and number of classes depends on the workload of classrooms and teachers.;
- evening time: 4 academic hours from 18:00 to 20:50 or from 19:00 to 21:50.
Teachers
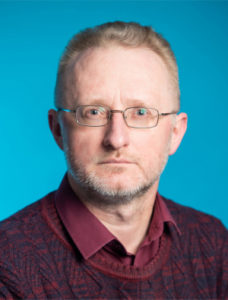
Candidate of Biological Sciences, Associate Professor, Principal Investigator, Department of Genetics, Belarusian State University. Molecular geneticist, bioinformatician, specialized in computational transcriptomics.
Phone: +375 (17) 209-58-60
E-mail: grinev_vv@bsu.by
End of course
To obtain a state-recognised professional development certificate, the student must successfully complete all the tasks of practical classes, as well as tasks intended for independent work. The final stage of training in this case is passing the test, where the task is to write a program code in R language and use it to analyze typical data.
Feedback
Vasily V. Grinev.
Office Address:
st. Kurchatov-10, office 425, Department of Genetics, Biological Faculty, Belarusian State University, 220010 Minsk, Belarus.
Official Address:
Department of Genetics, Biological Faculty, Belarusian State University, Nezavisimosti Avenue-4, 220050 Minsk, Belarus.
Work Phone: +375 (17) 209-58-60.
Viber: +375 (29) 188-16-93
E-mail: grinev_vv@bsu.by or grinev_vv@mail.ru
Instagram: https://www.instagram.com/biodataanalytics
Web-page: http://bio.bsu.by/genetics/grinev.html
YouTube channel “Grinev’s Educational Channel”: https://www.youtube.com/channel/UCYQ8QwQAX8ubVYYuegxNTYQ
Institute for Advanced Studies and Retraining of Belarusian State University.
E-mail: Klimova@bsu.by
Work Phone: +375 (17) 259-70-59.
Address: st. Kalvariyskaya-9, room 826, Minsk, Belarus.